Evaluating Effects of Linguistic Evolution on Classification Models for Banking Documents
Skills Employed
- Modelling Techniques: Classification Models - Random Forest, Logistic Regression, and Sparse Logistic Regression (l1-regularized)
- Cross Validation Techniques: Randomized Search CV (l1 penalty)
- Text Processing Techniques: Preprocessing (Lemmatization, Stop Word Removal, Removal of punctuation, numbers etc.), Keyphrase and keyword extraction
- Other Statistical Techniques: T-Test, Chi-squared test
- Tech Stack: Python, MS Excel
- Libraries: Numpy, Pandas, Sklearn, NLTK, Scipy, RAKE (python-rake)
Introduction
Linguistic Evolution can be broadly defined as the shift in word vocabulary, context and trends in a language in a particular area of interest. In 1968, Weinreich, Labov, and Herzog published a paper, Empirical foundations for a theory of language change to break this bigger area of study into 5 smaller problems. They are:
- The Actuation Problem: Why does a change in a particular feature take place in a particular language at a particular time, but not in other languages with the same feature, or in the same language at some other time?
- The Transition Problem: How does a language move from one state to another, and how are changes transmitted between speakers?
- The Embedding Problem: How is change embedded in linguistic structure and social structure?
- The Evaluation Problem: What role does social awareness play?
- The Constraints Problem: What changes are possible, and what factors constrain their likelihood?
In this project, we focussed on the Transition Problem. To explain further, this project was aimed to study the evolution of the vocabulary in banking documents like customer complaints and reviews and its correlation with the performance metrics (especially recall) of classification models built using these text documents itself. The hypothesis we tried to test here, was that as the complexity (difficulty of readability) of a language increases, the performance of the classification models improves (false-negatives decrease). The model for this particular case would predict mortgage payment faults. However, we anticipate the framework being scaled to other use-cases as well, with a few minor tweaks in the process described here. The detailed methodology followed in this project is depicted below.
Methodology
The detailed methodology followed in this project is explained below.
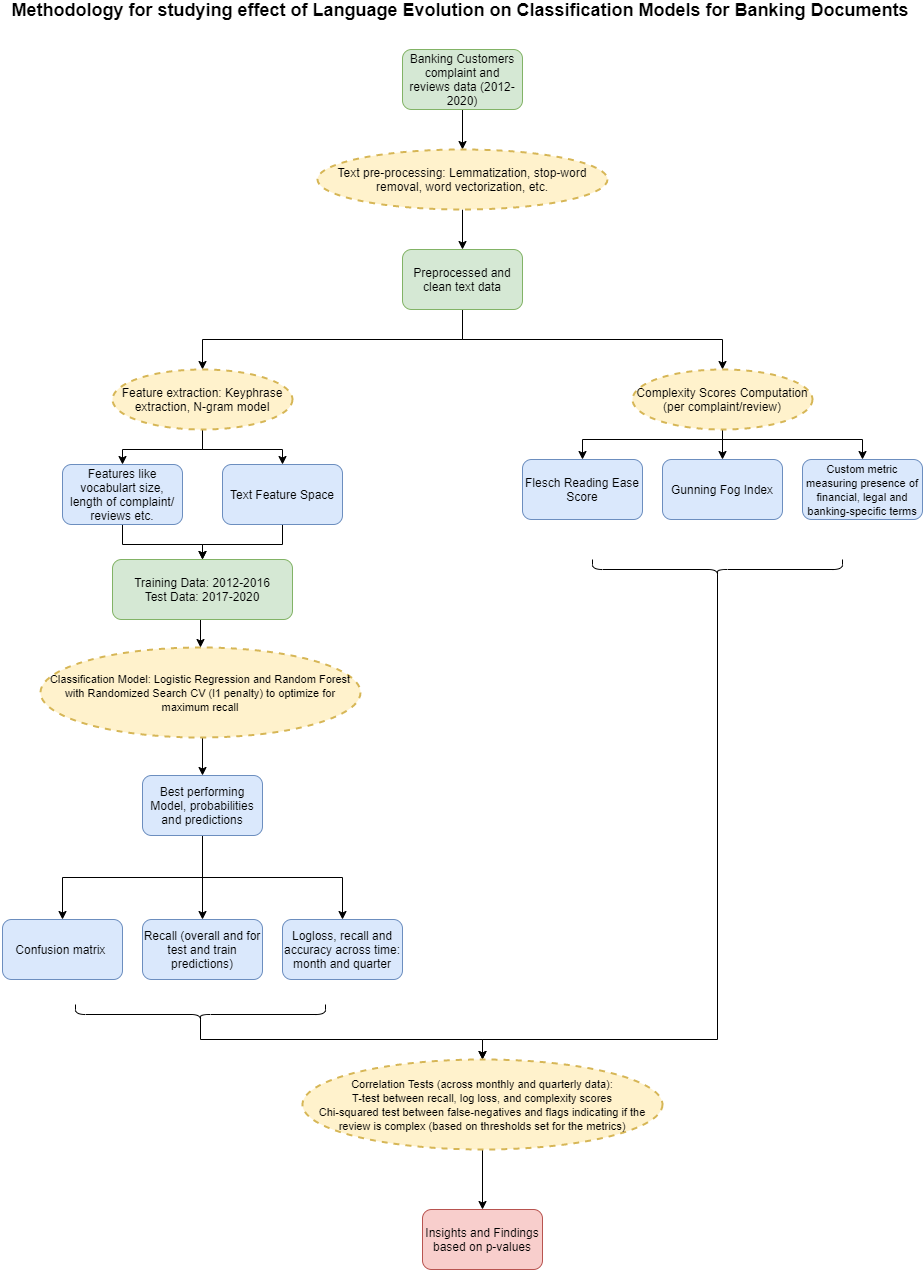
Impact
The model and framework described here were executed on a partly synthetic and partly deidentified actual data as it was completed as a part of a virtual internship with a banking company (Summer 2020). The framework and the models were then added to the research repository of the bank for further use in all such similar use-cases.
References
The following papers and literature were referred to, for execution and solution approach design.
- Roberts, G; Sneller, B; Empirical foundations for an integrated study of language evolution
- Hamilton, William L.; Leskovec, Jure; and Jurafsky, Dan; Cultural Shift or Linguistic Drift? Comparing Two Computational Measures of Semantic Change
- Weinreich, U; Labov, W; and Herzog, Marvin I.; Empirical foundations for an Theory of Language Change